Finding fraudsters: Algorithm predicts fraud with remarkable accuracy
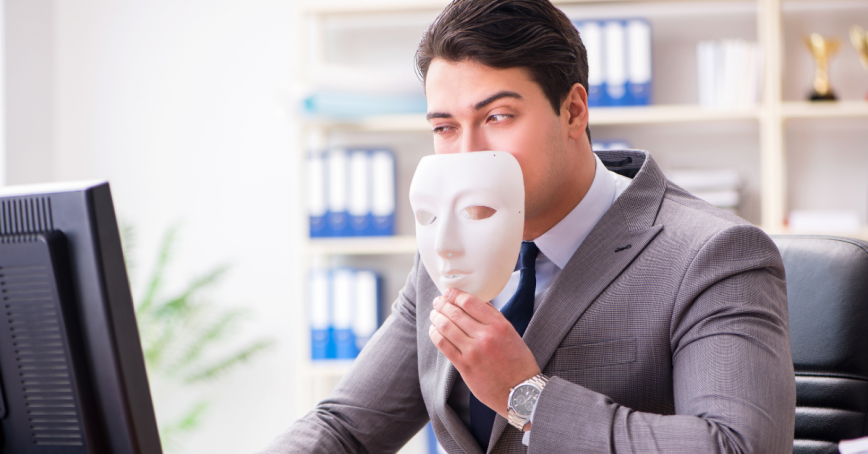
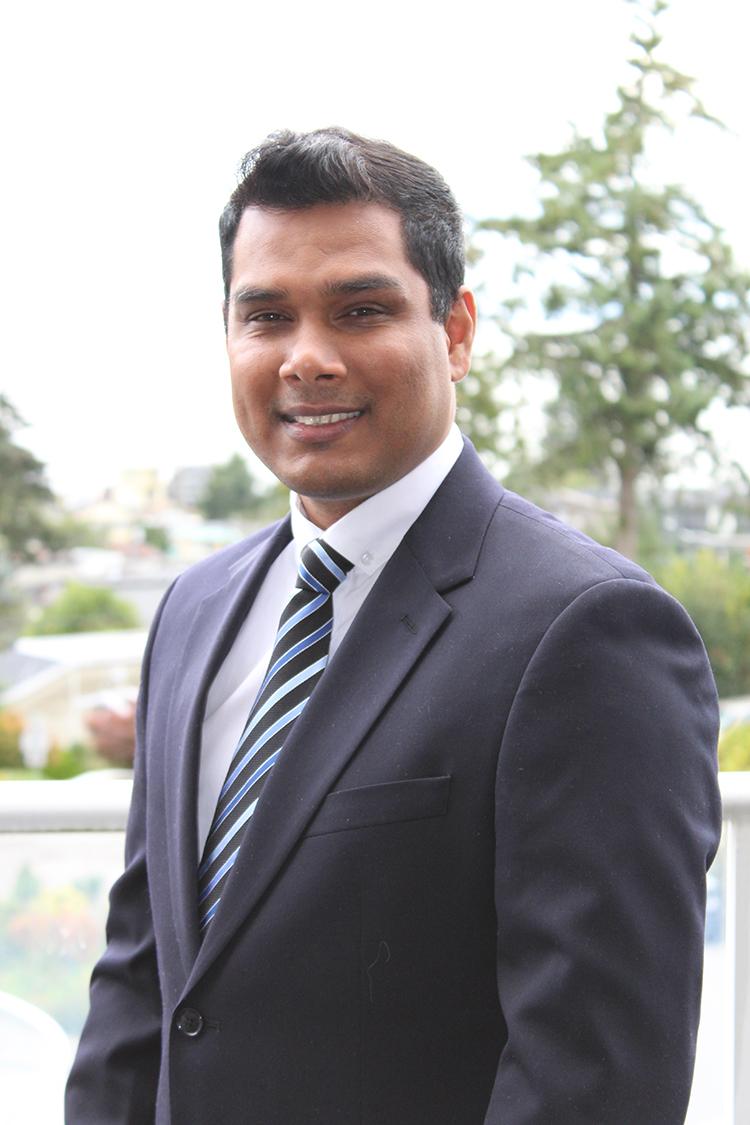
What kind of person commits financial fraud?
There’s a new algorithm for that thanks to School of Business Assoc. Prof. Mark Lokanan.
And it can pinpoint a fraudster with almost 100 per cent accuracy.
Lokanan and his team of researchers found the most common offences, 95 per cent, are regulatory breaches, such as discretionary and unauthorized trading.
More serious offences, such as swindling someone out of their life savings, make up five per cent.
The latter is what motivates Lokanan to build a better fraud detection model.
He was recently awarded an Insight Development Grant from the Social Sciences and Humanities Research Council of Canada for almost $52,000, as well as $4,000 from the university to investigate creating one self-regulatory organization to better protect investors and safeguard Canada’s capital markets.
Concerns have been raised that the Investment Industry Regulatory Organization of Canada (IIROC) and the Mutual Fund Dealers Association of Canada (MFDA) are not protecting investors and markets well enough. Those concerns resulted in calls to scrutinize the enforcement of complaints and to merge both organizations into a more powerful one.
The findings of Lokanan's project will be used to inform the consultation process on the streamlining of self-regulation in the Canadian financial markets and to inform the proposed consolidation between the two self-regulatory organizations.
Predicting fraud
As a data scientist, Lokanan employs machine learning techniques to build regression, classification and clustering algorithms to detect and investigate fraud. He built a fraud detection model to predict investment fraud using IIROC’s enforcement data that is now being turned into software for use by industry. It will also help shape the one he's building for the Mutual Fund Dealers Association (MFDA).
“I have to do the same thing. I have to build a model that can predict investment fraud based on MFDA data, and predict fines imposed by regulators on offenders,” says Lokanan, who previously worked as a fraud investigator for the Ministry of the Attorney General in Ontario.
“Before anything is determined, both the IIROC and MFDA will have insightful information to make decisions. Both the Ontario Securities Commission and the Canadian Securities Administrators will also have the information to make this decision, as well as the provincial governments, and the federal government, because this is a big merger. This is merging the two most important self-regulatory organizations in Canada.”
Building the IIROC model took three years. Lokanan and his team collected data from up to 900 files, then coded it for the most important features.
“We wanted to know what type of individuals will commit fraud, so we look at their employment background, we look at their years in the industry, we look at gender, age, we look at the type of people they're going to commit fraud against,” he adds.
They also look at the type of offences committed, from forgery to fraud and misappropriation of funds, and build a demographic profile.
“We input the variables into the model to predict the most important features that regulators and investment firms should look at in detecting red flags,” Lokanan says.
One would be an investment advisor, working on commission, trading in a client's account without signatory authorization.
Unauthorized trading
Lokanan found many investment advisors were arbitrarily trading on behalf of people without that signature, consent or prior knowledge.
“A lot of these trades result in losses,” he says. “Fraud, forgery, misappropriation of funds, those are criminal offences.”
But discretionary and unauthorized trading are classified as regulatory offences that Lokanan argues should be classified as criminal offences.
“You cannot prosecute regulatory offences in criminal courts. They go to a tribunal. You will probably get a fine or a suspension.”
Lokanan says his model has predicted such fraud with 99.9 per cent accuracy.
“We try four or five algorithms, then we look at the one with the highest accuracy of predicting fraud.”
While Lokanan says such algorithms have existed for decades, the kind of computational speed and technology necessary to use them right, hasn’t.
“Now with cloud technology, with more sophisticated computers, we can run these models in less time than it would have taken us 10 years ago,” he adds.
Over the next 18 months or so, Lokanan will use the same codes to build a new algorithm to detect fraud in the MFDA dataset.
It is expected that other researchers can build on his approaches to develop new theories and framework that will inform sanction guidelines and disciplinary mechanisms.
“One of the main criteria for the grant was how I will pass on my knowledge to the next generation of fraud fighters,” he says. “The driver is to protect the public interest, protect investors, protect hardworking Canadians from being swindled by unscrupulous investment advisors.”